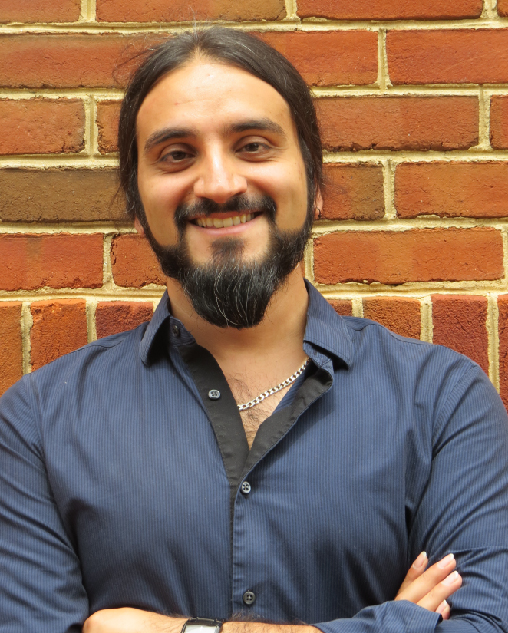
Babadi, Behtash
Associate Chair for Graduate Studies
The Institute for Systems Research
Brain and Behavior Institute
Behtash Babadi received the Ph.D. and M.Sc. degrees in Engineering Sciences from Harvard University in 2011 and 2008, respectively, and the B.Sc. degree in Electrical Engineering from Sharif University of Technology, Tehran, Iran in 2006. From 2011 to 2014, he was a post-doctoral fellow at the Department of Brain and Cognitive Sciences at Massachusetts Institute of Technology as well as at the Department of Anesthesia, Critical Care and Pain Medicine at Massachusetts General Hospital. His research interests include statistical and adaptive signal processing, neural signal processing, and systems neuroscience.
Education
Ph.D. in Engineering Sciences, Harvard University, 2011
M.Sc. in Engineering Sciences, Harvard University, 2008
B.Sc. in Electrical Engineering, Sharif University of Technology, Tehran, Iran, 2006
Honors and awards
E. Robert Kent Teaching Award for Junior Faculty (2019)
NSF Faculty Early Career Development (CAREER) Award (2016)
GSAS Merit Fellowship, Harvard University (2010)
Statistical and adaptive signal processing, neural signal processing, systems neuroscience
- A. Sheikhattar, S. Miran, J. Liu, J. B. Fritz, S. A. Shamma, P. O. Kanold, and B. Babadi, Extracting Neuronal Functional Network Dynamics via Adaptive Granger Causality Analysis, Proceedings of the National Academy of Sciences, Vol. 115, No. 17, E3869-E3878, April 2018.
- S. Miran, S. Akram, A. Sheikhattar, J. Z. Simon, T. Zhang, and B. Babadi, Real-Time Tracking of Selective Auditory Attention from M/EEG: A Bayesian Filtering Approach, Frontiers in Neuroscience, Vol. 12, pp. 262, May 2018.
- N. A. Francis, D. E. Winkowski, A. Sheikhattar, K. Armengol, B. Babadi, and P. O. Kanold, Small Networks Encode Decision-Making in Primary Auditory Cortex, Neuron, Vol. 97, No. 4, Feb. 21, 2018.
- P. Das and B. Babadi, Dynamic Bayesian Multitaper Spectral Analysis, IEEE Trans. on Signal Processing, Vol. 66, No. 6, pp. 1394-1409, March 2018.
- A. Kazemipour, J. Liu, K. Solarana, D. A. Nagode, P. O. Kanold, M. Wu, and B. Babadi, Fast and Stable Signal Deconvolution via Compressible State-Space Models, IEEE Trans. on Biomedical Engineering, Vol. 65, No. 1, pp. 74-86, Jan. 2018.
- S. Miran, P. L. Purdon, E. N. Brown, and B. Babadi, Robust Estimation of Sparse Narrowband Spectra from Neuronal Spiking Data, IEEE Trans. on Biomedical Engineering, Vol. 64, No. 10, pp. 2462-2474, Oct. 2017.
- A. Sheikhattar, J. B. Fritz, S. A. Shamma, and B. Babadi, Recursive Sparse Point Process Regression with Application to Spectrotemporal Receptive Field Plasticity Analysis, IEEE Trans. on Signal Processing, Vol. 64, No. 8, pp. 2026—2039, April 2016.