News Story
ECE Names 2024-2025 Distinguished Dissertation Fellows
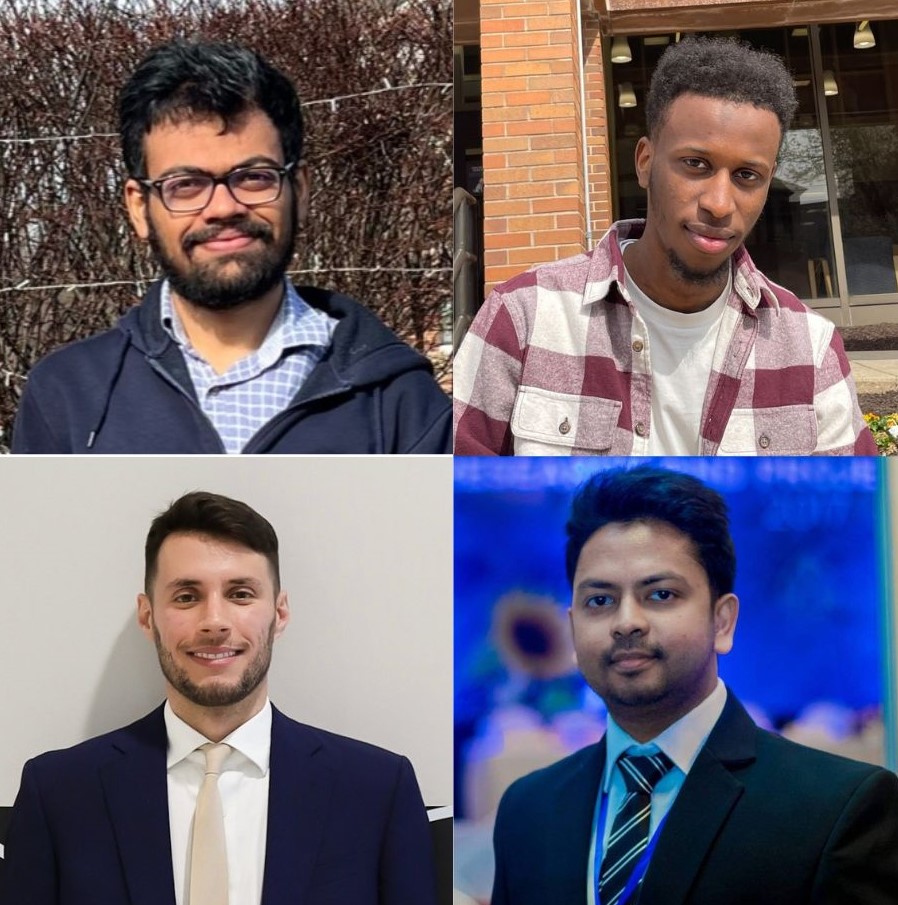
Clockwise from top left: Subhankar Banerjee, Faisal Hamman, Zachary Lazri, Kasun Weerakoon
The Department of Computer and Electrical Engineering (ECE) recently named their 2024-2025 Distinguished Dissertation Fellowship awardees. The Distinguished Dissertation Fellowship is a departmental award recognizing outstanding students in the final stages of their dissertation work and seeks to provide both a financial award and recognition of the student's research excellence. The following author’s dissertations were selected by a search committee comprised of ECE faculty members Richard La, Jonathan Simon, Cunxi Yu and Kevin Daniels.
Subhankar Banerjee
Advised by Professor Sennur Ulukus
Dissertation Title: Optimal Control in Status Update Systems
Recent advancements in communication technologies have paved the way for a wide range of cutting-edge applications, including self-driving cars, industrial and home IoT systems, and real-time augmented and virtual reality (AR/VR). A unifying characteristic among these applications is their critical dependence on timely communication and the continuous availability of fresh information. Ensuring low latency and data freshness is essential to maintain performance, reliability, and safety in such systems. Banerjee’s thesis focuses on optimizing information freshness across various network models. By developing and analyzing strategies tailored to different scenarios, he aims to improve the timeliness and efficiency of modern communication systems that support these emerging technologies.
Faisal Hamman
Advised by Professor Sanghamitra Dutta
Dissertation Title: Trustworthy and Explainable Machine Learning Using Information Theoretic Methods
Motivated by the growing demand for trustworthy and explainable/interpretable machine learning (ML) models in high-stakes domains such as finance, healthcare, and education, Hamman's research leverages information-theoretic methods to tackle key challenges in explainability, robustness, and the reliability of ML models in these critical settings. His work focuses on developing mathematical frameworks that ensure ML systems provide reliable and actionable explanations for their predictions, maintain robustness in the face of model changes, and ensure consistent and stable outputs in large language models (LLMs). His research aims to improve the transparency and safe deployment of artificial intelligence (AI) systems, fostering trust in critical applications.
Zachary Lazri
Advised by Professor Min Wu
Dissertation Title: Analyzing and Enhancing Algorithmic Fairness in Social Systems and Data-Restricted Applications
Artificial intelligence (AI) and machine learning (ML) increasingly influence high-stakes decisions in high stakes applications, such as finance, healthcare, and education. As their reach grows, so do concerns about their potential to reinforce social inequities. Lazri’s research addresses this challenge by rethinking how fairness is defined, modeled, and evaluated, ensuring that these systems serve the public good. His work begins with the development of a formal definition of within-group fairness, addressing the fact that models can treat individuals unfairly even when group-level fairness is achieved. He proposes a preprocessing technique that ensures both inter- and intra-group fairness while preserving individuals’ relative standing, particularly under real-world constraints where sensitive attributes may be unavailable. Building on this, he introduces a framework for evaluating trade-offs between fairness and accuracy under data restrictions, revealing how tensions depend on both fairness definitions and dataset structure. These findings point to a deeper issue: many fairness formulations assume static, one-shot decisions, overlooking how real-world disparities emerge over time. To address this, he develops Multi-Agent Fair Environments (MAFEs)--simulation-based testbeds that model how fairness evolves in dynamic, multi-agent systems. By addressing fairness across individual, group, and systemic levels, his research contributes perspectives for building more equitable AI systems.
Kasun Weerakoon Kulathun Mudiyanselage
Advised by Professor Dinesh Manocha
Dissertation Title: Towards Fully Autonomous Robot Navigation: A Multi-Modal Perception and Learning-Based Approach
Weerakoon’s dissertation advances autonomous navigation for mobile robots in complex outdoor environments through a multi-modal perception and learning-based framework. Complex outdoor environments present a range of challenges, including uneven terrain, dense vegetation, degraded or partial sensing, and dynamically evolving, context-rich scenarios that demand robust, adaptive, and intelligent decision-making capabilities. His dissertation research addresses these challenges through three core contribution domains. First, it introduces learning-based navigation policies designed for real-world deployment. These include both online and offline deep reinforcement learning (DRL) methods that fuse multiple sensory modalities to enable stable, efficient navigation, even under sparse reward conditions. Second, it enhances perception robustness in the presence of degraded or incomplete sensing. This is achieved through novel traversability estimation algorithms and a new 3D representation that supports semantic and geometric reasoning in cluttered, unstructured terrain. Third, it enables high-level, context-aware navigation by integrating compact vision-language models. This allows robots to interpret natural language instructions and environmental context, supporting behavior-aware planning in open-world settings. Collectively, these contributions result in flexible, scalable, and interpretable navigation systems with strong potential for real-world deployment in diverse field robotics applications.
Published May 23, 2025