News Story
Shah Receives NSF Grant to Improve Extreme Edge Devices
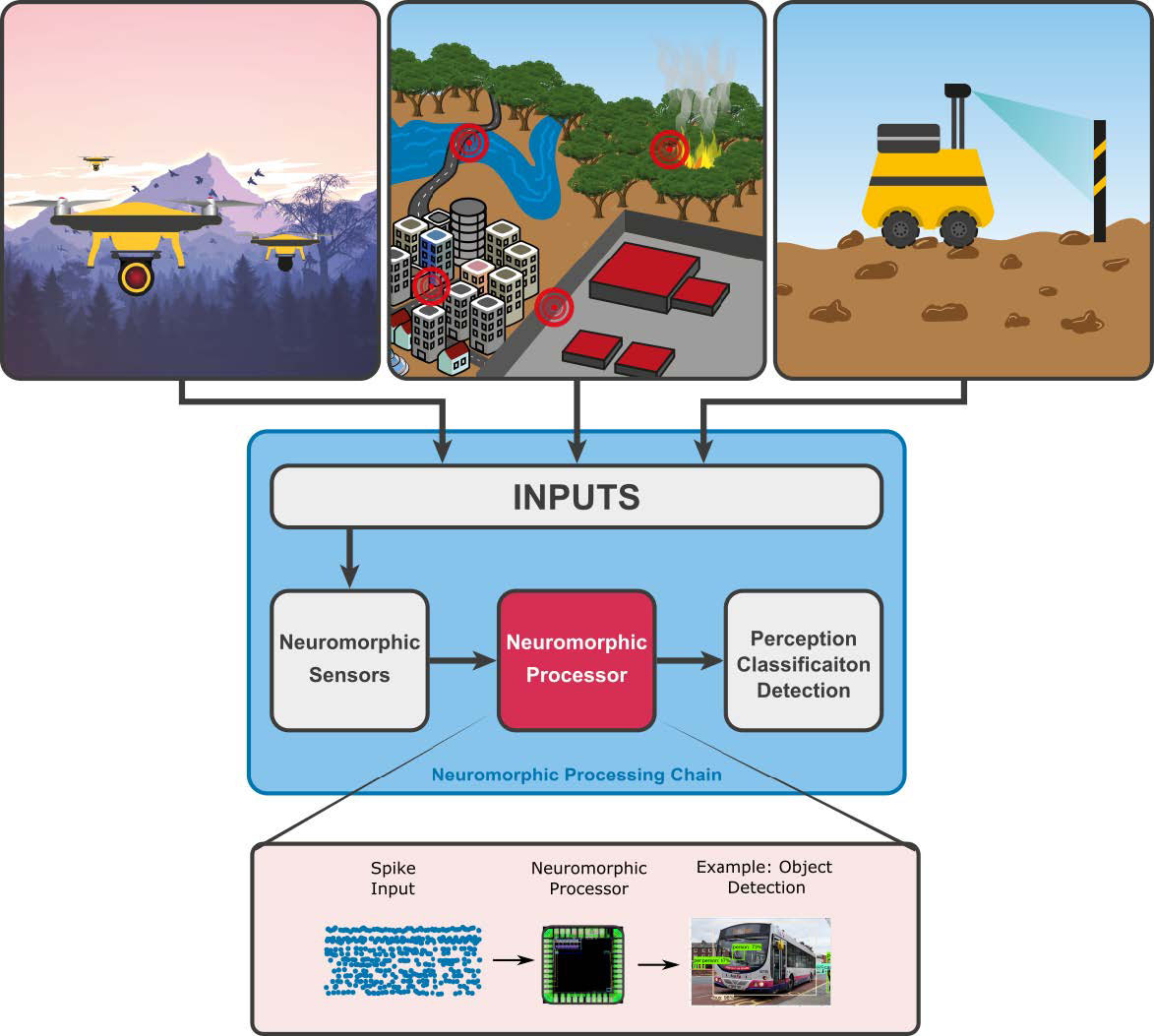
Potential applications where extreme edge neuromorphic processors (highlighted in red) can enable always-on monitoring in remote locations. Depending on the application the reconfigurable neuromorphic processor can be configured to perform tasks with different complexity. For instance, a neuromorphic processor on a drone can detect objects in its path.
Assistant Professor Sahil Shah has been awarded a three-year, $336,197 National Science Foundation (NSF) Grant as Principal Investigator to develop"Reconfigurable Neuromorphic Computing to enable Energy-Efficient Edge Intelligence". The goal of the project is to develop an energy-efficient and smart extreme edge device by taking inspiration from the human brain.
Extreme edge devices transmit data from local networks to the cloud and have a number of uses, such as remote tracking of rapid changes in the arctic, autonomous navigation of aerial and ground robots for deep space exploration, and monitoring and securing critical infrastructure. However, there are some limitations to the current technology, which include a delay between sensing and decision, shorter battery life due to high power consumption and privacy concerns related to data transfer.
With this grant, Dr. Shah intends to develop hardware that will address these limitations and advance the development of an extreme edge device that maintains a reconfigurable brain-inspired processor with the ability to learn based on input date. Additionally, he aims at developing this hardware using open-source computer-aided design tools to enable broader dissemination of the work and also to train new generations of students in microelectronics.
Shah joined the ECE Department in Spring 2021. Previously, he was a postdoctoral associate in the department of Electrical Engineering at California Institute of Technology, where his principal research focused on developing brain-machine interface for enabling patients to control prosthetic devices. He is continuing his research at UMD with a particular focus on energy-efficient integrated circuits, embedded machine learning and bio-sensing and monitoring.
Published August 31, 2022