News Story
Maryland researchers develop computational approach to understanding brain dynamics
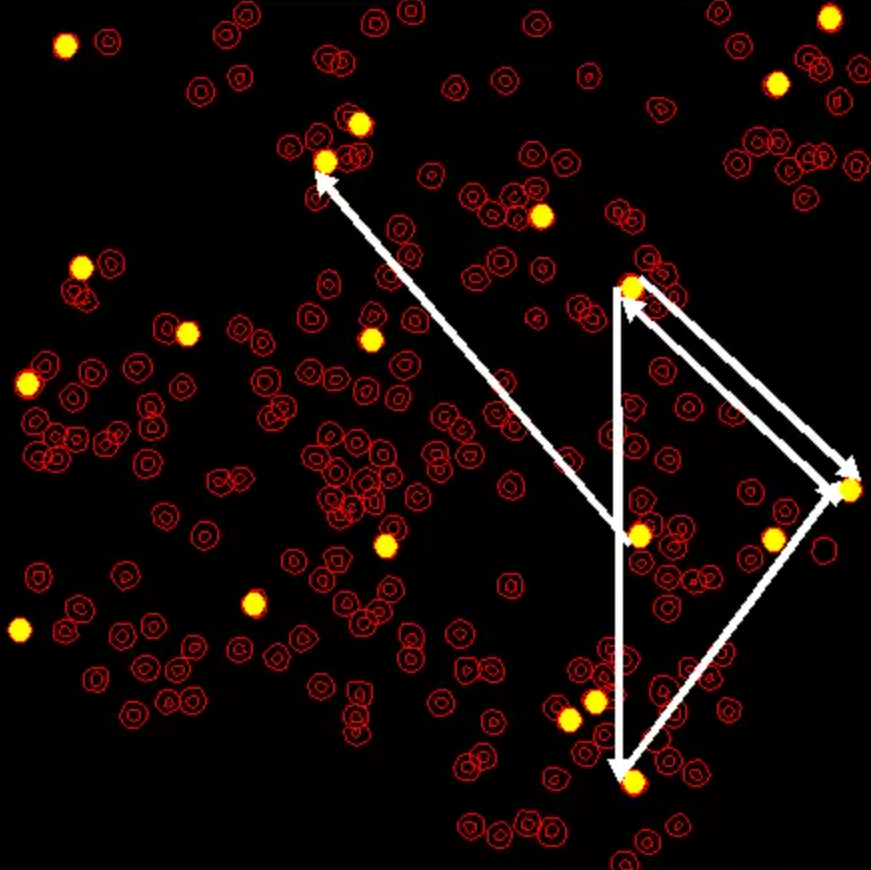
Brain neuron network mapping (see the video below).
Scientists often refer to the human brain as the most complicated biological structure on Earth; perhaps the most complex object in the universe. The quest to understand how the brain functions, by mapping it down to the neural level, has proven to be a much larger and more complex project than mapping the human genome. Neuroscientists have been working on this problem for more than a century.
One approach has been to try to work out the brain’s “connectome,” the roadmap of all the connections of all the nearly 100 billion neurons in the human brain, in the hope that this structural map will give insight to how the brain works. However, the brain is a highly plastic structure that can adaptively change as it meets new challenges.
Now, research by a team of University of Maryland theoreticians and experimentalists just published in the Proceedings of the National Academy of Sciences of the United States of America (PNAS) provides a new computational approach to understanding the brain that probes the dynamic and changing functional interactions between the nodes in its neuronal networks. Their paper, “Extracting neuronal functional network dynamics via adaptive Granger causality analysis,” was written by Assistant Professor Behtash Babadi (Electrical and Computer Engineering), his graduate students Alireza Sheikhattar and Sina Miran, Professor Patrick Kanold (Biology), his graduate student Ji Liu, Professor Shihab Shamma (ECE/Institute for Systems Research) and Research Scientist Jonathan Fritz (ISR).
Led by Babadi, the team developed new signal processing techniques that extract functional network dynamics from neuronal data. These techniques then were used to study changes in neural functional networks during attentive behavior in both mice and ferrets. They were applied to two-photon calcium imaging data from the mouse auditory cortex in collaboration with Kanold and to simultaneous recordings from neurons in the ferret auditory and prefrontal cortices in collaboration with Shamma and Fritz.
The new approach goes beyond the connectome, showing that complex networks can be dynamically re-organized in multiple functional configurations that best suit the task the brain is engaged in at that moment. In addition, the collaboration between theoreticians and experimentalists shows the promise and value of neuroengineering, an interdisciplinary fusion that will lead to further advances in the understanding of how the brain functions.
| Learn more and view additional material at the PNAS website |
Videos
The above media file shows the network maps for the entire data duration of ∼22 min (1,333 s) overlaid on the slice (four snapshots shown in Fig. 5D). Neurons are shown with red circles and the selected 20 neurons are highlighted in yellow, scattered across the whole slice. The white directed arrows represent the detected G-causal links, where the width of each arrow at each time reflects its significance level (i.e., its corresponding J-statistics). The horizontal cursor at the bottom represents time in seconds.
The above media file exhibits the STRF estimates of all of the five A1 units for the entire experiment duration (three snapshots shown in Fig. 6D). The excitatory and inhibitory effects are shown with red and blue colors, respectively. Each STRF panel is a 50×50 array, with I=50 lag bins uniformly spanning time lags in the range of [0,50] ms on the horizontal axis, and J=50 frequency bins spanning the frequencies in the range of [500,16,000] Hz in logarithmic scale on the vertical axis. The horizontal cursor at the bottom represents time in seconds.
Published April 10, 2018