News Story
Wired Magazine Features Ott and Collaborators’ work on Machine Learning to Predict Chaos
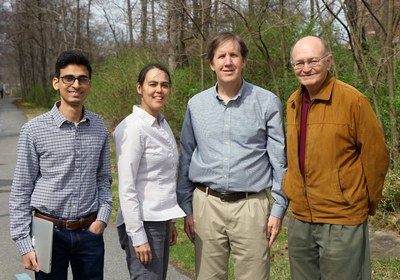
Distinguished University Professor Edward Ott (ECE/Physics/IREAP) and collaborators at the University of Maryland have been featured in an article in Wired Magazine.
The article, originally published in Quanta Magazine and reprinted in Wired, titled “Machine Learning’s ‘Amazing’ Ability to Predict Chaos,” details the results of new papers published in the journals Physical Review Letters and Chaos, to highlight the application of machine learning to calculate the future evolution of chaotic systems out to extremely distant horizons.
The interdisciplinary researchers include Prof. Ott and current graduate student and lead author on the paper Jaideep Pathak, Associate Professor Michelle Girvan (IPST/Physics), Professor Brian Hunt (IPST/Mathematics), and Postdoctoral Researcher Zhixin Lu (now of the University of Pennsylvania).
The researchers’ employed a machine-learning algorithm termed reservoir computing to “learn” the dynamics of an archetypal chaotic system called the Kuramoto-Sivashinsky equation, and the evolving solution to this equation acts like a flame front, flickering as it advances through a combustible medium.
Their results demonstrate that machine learning, and more specifically reservoir computing, offers an effective potential means for model-free prediction of large spatiotemporally chaotic systems. Their technique can be applied to areas such as weather forecasting and monitoring of cardiac arrhythmias for signs of approaching heart attacks, as well as neuronal firing patterns in the brain for signs of neuron spikes.
Read the Quanta Magazine article by Natalie Wolchover here.
Published May 2, 2018